What is Artificial Intelligence (AI)? Is it robots taking over? Is it humans being displaced of white-collar jobs that were regarded as immune to automatization? Well, in my view, it's none of those—at least not for now. AI, more precisely, Applied-AI, is best understood as a tool to transform business models.
My career is in business, in the investment sub-segment of finance. I started back in 2000 as an investment banker in New York being involved in IPOs, acquisitions, mergers, spin-outs, and bond offerings, among others. Then, in 2012, I transitioned to Private Equity and worked for one of the largest investment firms in the world; a friend of mine accurately described it as being paid to worry as an owner (he is a large business owner himself). As a board member in six companies, I understood the importance of understanding the moats in each. The next task was selecting the right CEO and management team to design and execute the optimal strategy to create the most value and produce robust returns for our investors.
"Is it humans being displaced of white-collar jobs that were regarded as immune to automatization? Well, in my view, it's none of those—at least not for now. AI, more precisely, Applied-AI, is best understood as a tool to transform business models”
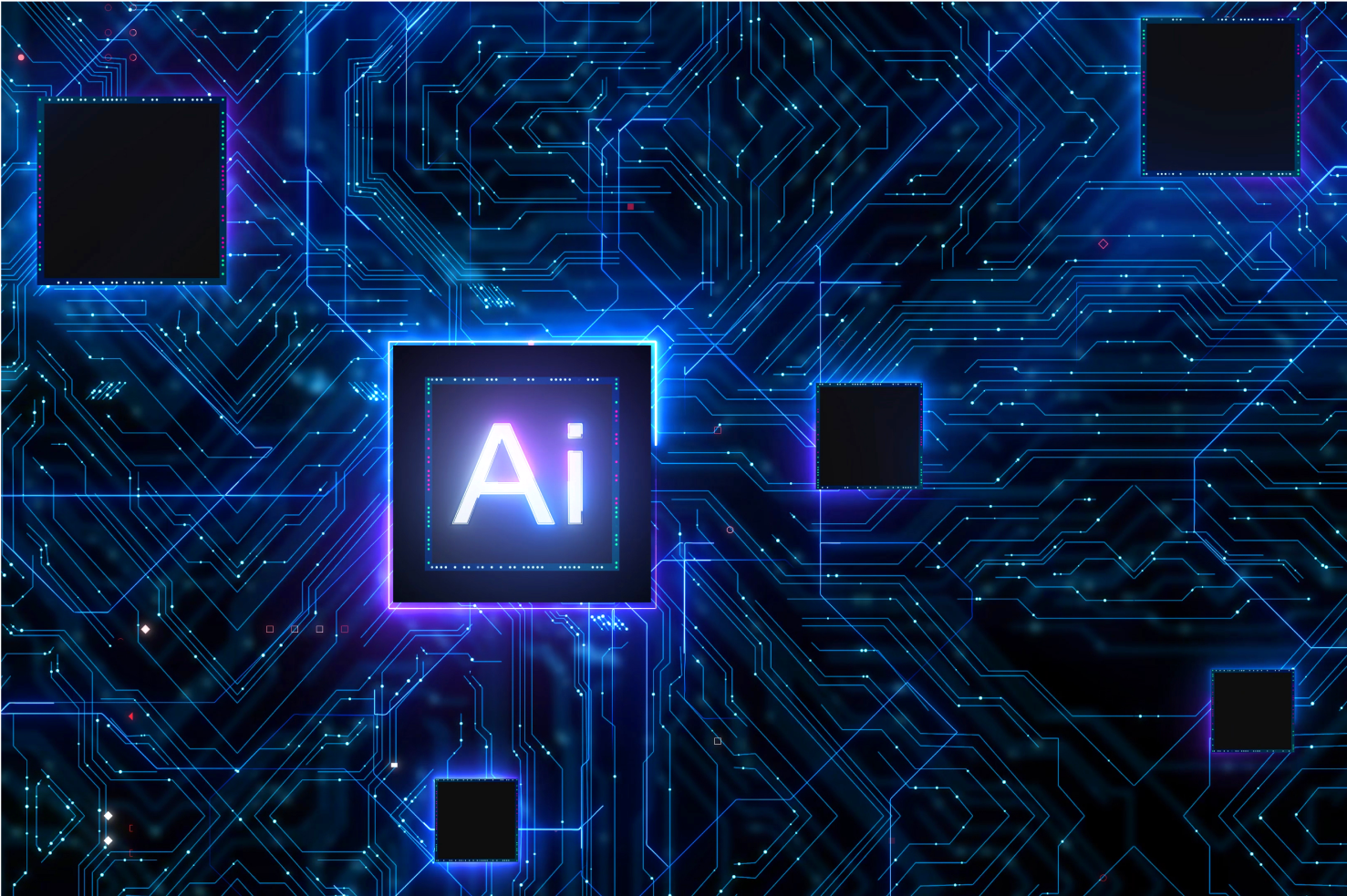
Nowadays, I operate in the realm of startups, as an angel investor in companies that are developing and deploying AI in different industries. Some examples include startups using computer vision to detect skin diseases, LiDAR to optimize forest management processes, AI models to optimize (maximize) the durability of biomaterials replacing plastics, or self-learning models used to maximize gross profit for small e-commerce businesses. If you think this is a small industry, think again. McKinsey, in its Technology Trends Report, issued August 2022, argues that the potential value at stake from AI is $10T to $15T; coming mostly from marketing and sales; supply chain management and manufacturing; and, to a lesser extent, service operations, product development, risk, and, strategy and corporate finance. VC investors are also noticing this trend and are deploying capital into AI-driven startups. As of December 2022, CB Insights shows 90 global unicorns in AI worth an aggregate of $342B.
It is from this vantage point that I would like to discuss the revolution of AI and explain why there is an opportunity to apply it as a tool to drive productivity, to create insurmountable moats, and therefore, act as an enabler to create and capture value.
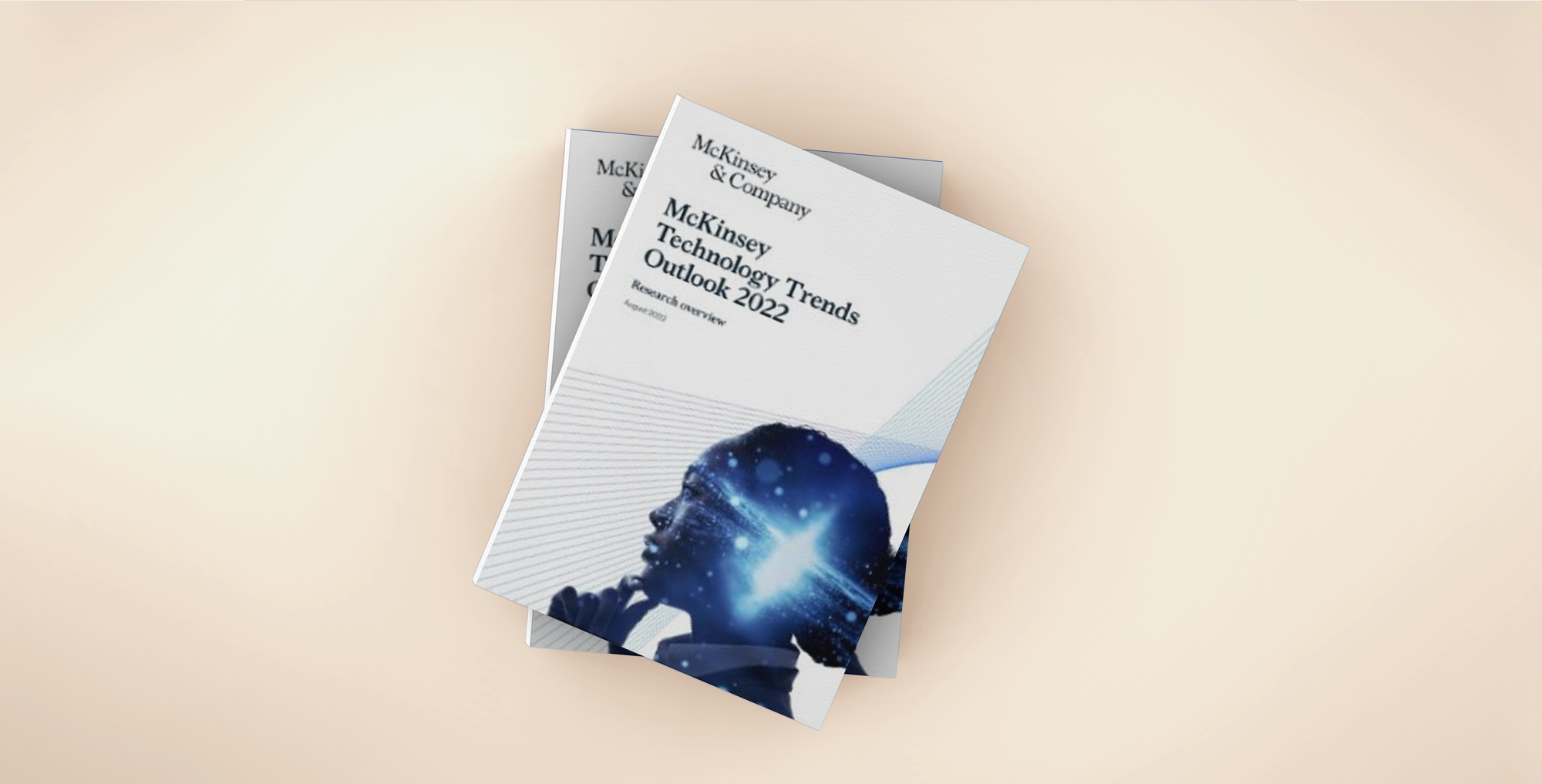
McKinsey Technology Trends 2022
Which technology trends matter most for companies in 2022? New analysis by the McKinsey Technology Council highlights...
The best analogy I have heard is to equate AI to electricity. Andrew Ng made this argument in 2017 in Why AI is the New Electricity and said: “Just as electricity transformed everything 100 years ago, today I actually have a hard time thinking of an industry that I don’t think AI will transform in the next several years.” To clarify, it was the application of electricity in industrial processes that generated massive productivity gains, allowing us to produce more with fewer resources; factories no longer had to locate themselves next to a river for power.
So, why is AI the new electricity? For that, I found my answer in a book titled Prediction Machines by Ajay Agrawal, Joshua Gans, and Avi Goldfarb; all of whom operate in the intersection of AI and economics. I highlight two arguments from their work. The first is that AI is augmenting one component of intelligence: prediction. The second is that computational power has made the application of AI (or prediction) cheap and therefore abundant.
AI models can predict an output based on several inputs. Put simply, AI is based on statistical models and regression models are one of the most basic ones. These models gather data and then draw a line that best fits that data (in math terms, minimize the squared-error) and then produce a “model” that says based on these inputs (“x”) this is the most likely output (“y”). Of course, AI carries this concept to higher levels of complexity beyond humble regressions, but this is the essence.
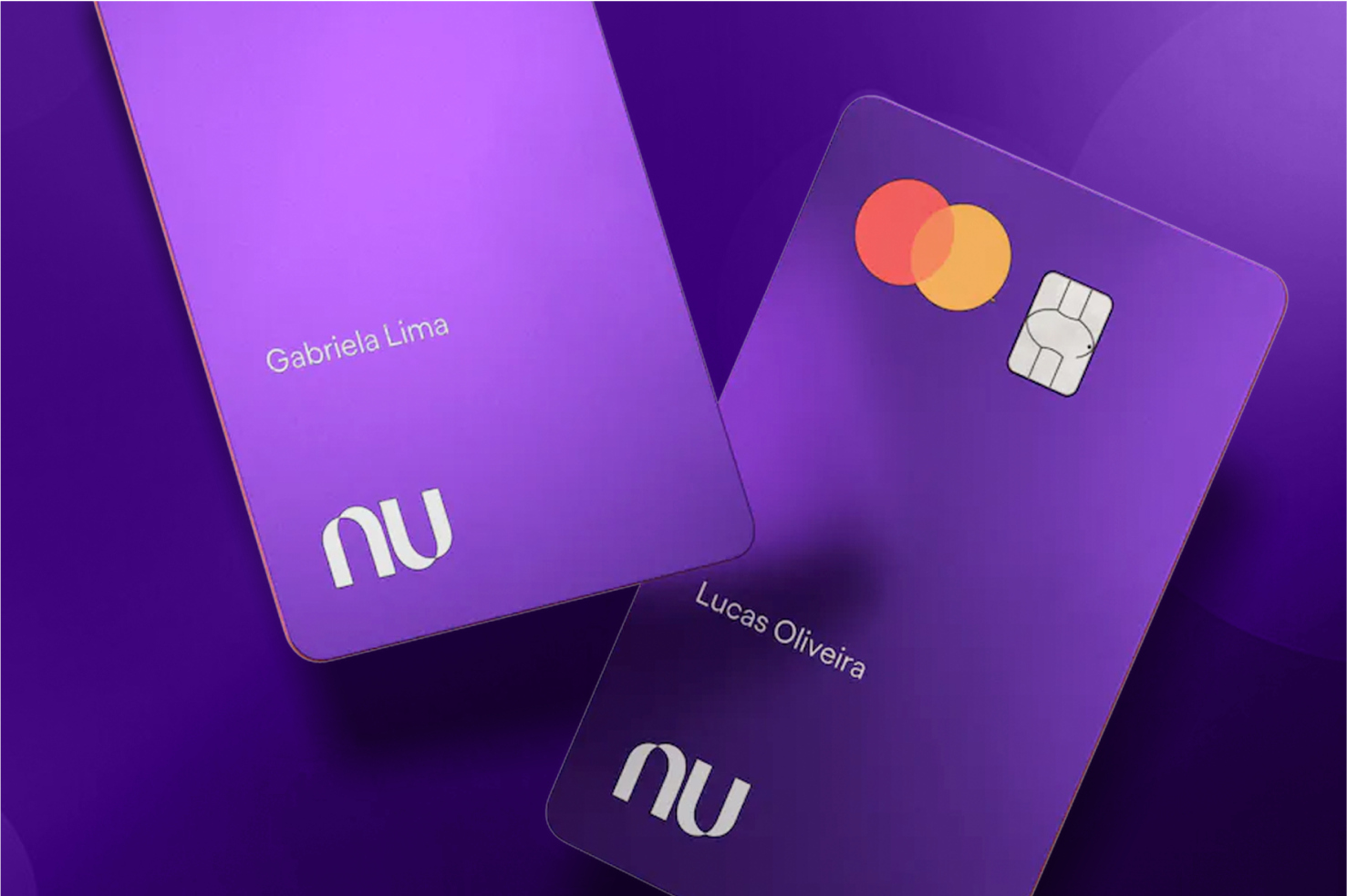
Let me offer an example. We have all seen Nubank —the largest fintech bank in Latin America backed by Warren Buffet— become a success. Nubank started in Brazil, a country with well-established traditional banks. This neobank uses AI to predict the repayment probability of issuing a loan to the unbanked. I have no inside knowledge of their exact model, but I imagine it went along these lines: it started by gathering available data on individuals with available credit history, such as prepaid cell phone charges, traffic on their business location, amount of inventory, et cetera, and used analytics to understand the association (more precisely the correlation) between these variables and their credit scores. Put simply, this AI model uses data we have (behavior of the banked) to produce data we don’t have (repayment probability of the unbanked). When someone without a credit history applies for a loan, the interface (via a mobile phone) requests the input data and, with it, runs their model to predict a credit score. This is the prediction component. Then —and here is the novelty— issues a small, short loan (say $10 for 6 weeks) to test this prediction. After 6 weeks, the loan is repaid (or not) and the model now has new and proprietary data. This feedback makes the model better with each iteration; a virtuous cycle that creates network effects. Further, it can scale as the applicant is now offered a new and larger loan, and this test is run again. Why is this insightful? Well, because the business development expenses relied initially on only risking $10 to gather valuable data that previously did not exist in an unattended market segment. This is the alternative to spending the traditional upfront or fixed costs a bank usually incurs: CapEx for a branch and salaries for loan officers. I have to clarify that the model does the prediction, but the judgment of issuing the loan above a certain score (and to increase the amount of the second loan) is done by a human applying her or his business acumen. This is an important distinction. AI models can process huge amounts of data, uncover hidden correlations, and tell us that “y” happens when “x” is present, but the human decides what to do with that information. AI models don’t make judgment calls, they augment or automate processes based on the information we feed them.
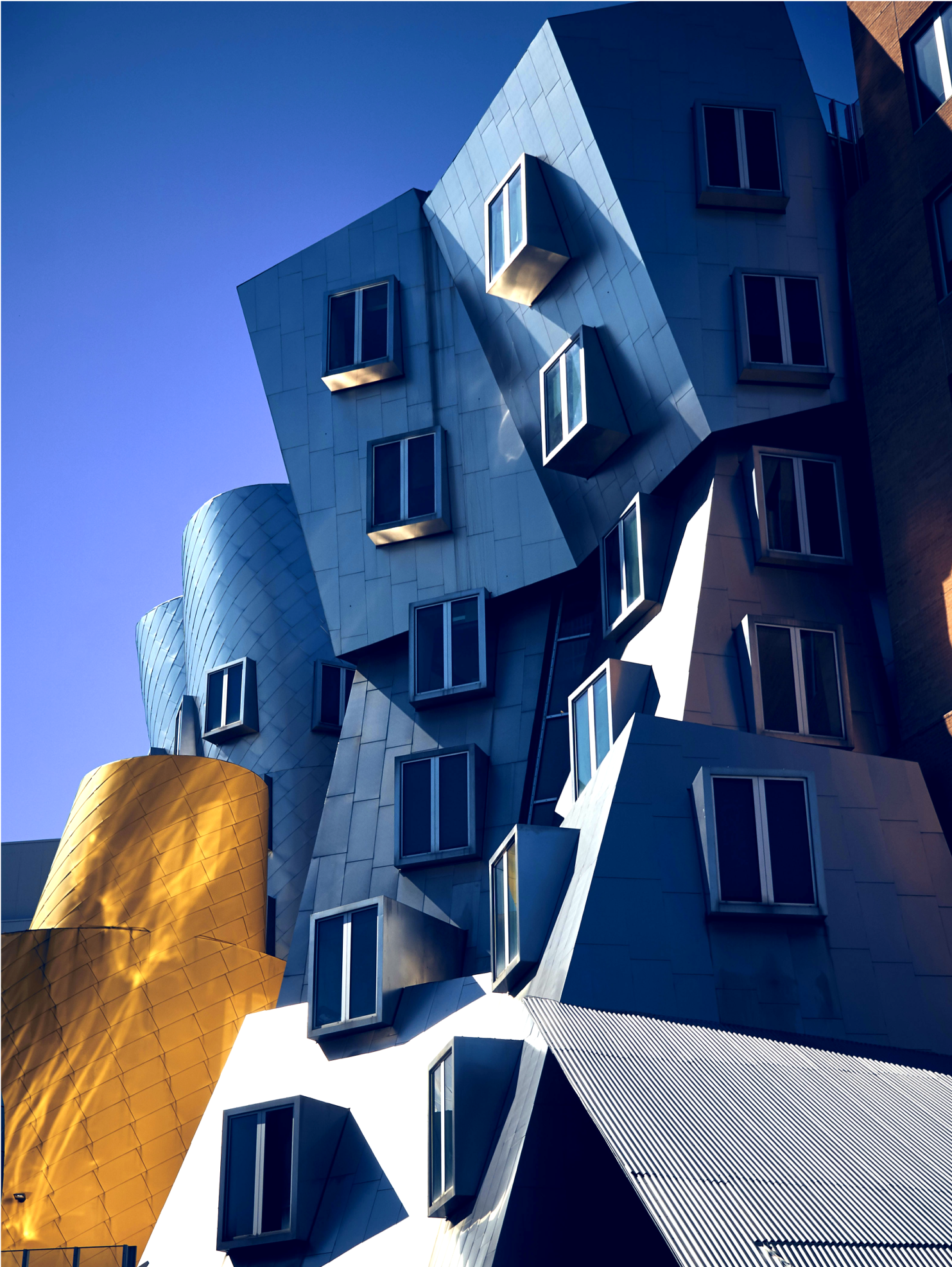
Another example that MIT Professor Alexis Montecinos makes in his class is that an AI model can individualize decisions on a massive scale. To exemplify this, he describes a situation where an online retail business does not want to issue a blanket promotion, such as a 20% discount on certain items, but instead wants to find a specific discount that will trigger a specific customer at a specific point in time to purchase a specific product. The AI model can learn this by sending first a 5% discount, then a 10%, then a 15%, until that customer clicks and buys. For another customer, this threshold might be 25%, and for another 5%. The point here is that the business can automate a process to learn customer habits and maximize gross profits, applied to thousands of items concurrently. It can do this automatically and under scenarios unmanageable by a marketing team. For example, it can “know” that for me, I’m most likely to click on a discount on a trip abroad before my daughter’s 16th birthday and on a Saturday when I’m not distracted by work (the system does not know this, but based on my prior behavior and a few intelligent tests, the system can predict a higher likelihood of purchase on an individual basis).
I used both examples to show the magnitude of productivity gains that can be achieved when AI is strategically applied in businesses.
The second component of how AI’s impact on business is growing and will continue to grow is how cheap AI has become. This also means it is now widely available and abundant. With the advent of both cloud computing and faster servers (GPUs have proven particularly great to run AI models), the cost of running models is surprisingly affordable. If you want to play around with an image classification model for free, try Teachable Machine by Google. The point is, that you don’t need to buy high-performing servers, hire an IT team, and deal with the traditional complexities of implementing new technologies to access the benefits. This is both an opportunity and a threat because it is very likely that your competitor is already thinking about establishing a business analytics team, or has hired a Chief Data Scientist. There could also be a startup that can move fast and capture this value. Those that neglect this reality will have a hard time staying competitive. It’s similar to Kodak neglecting the invention of the digital camera.
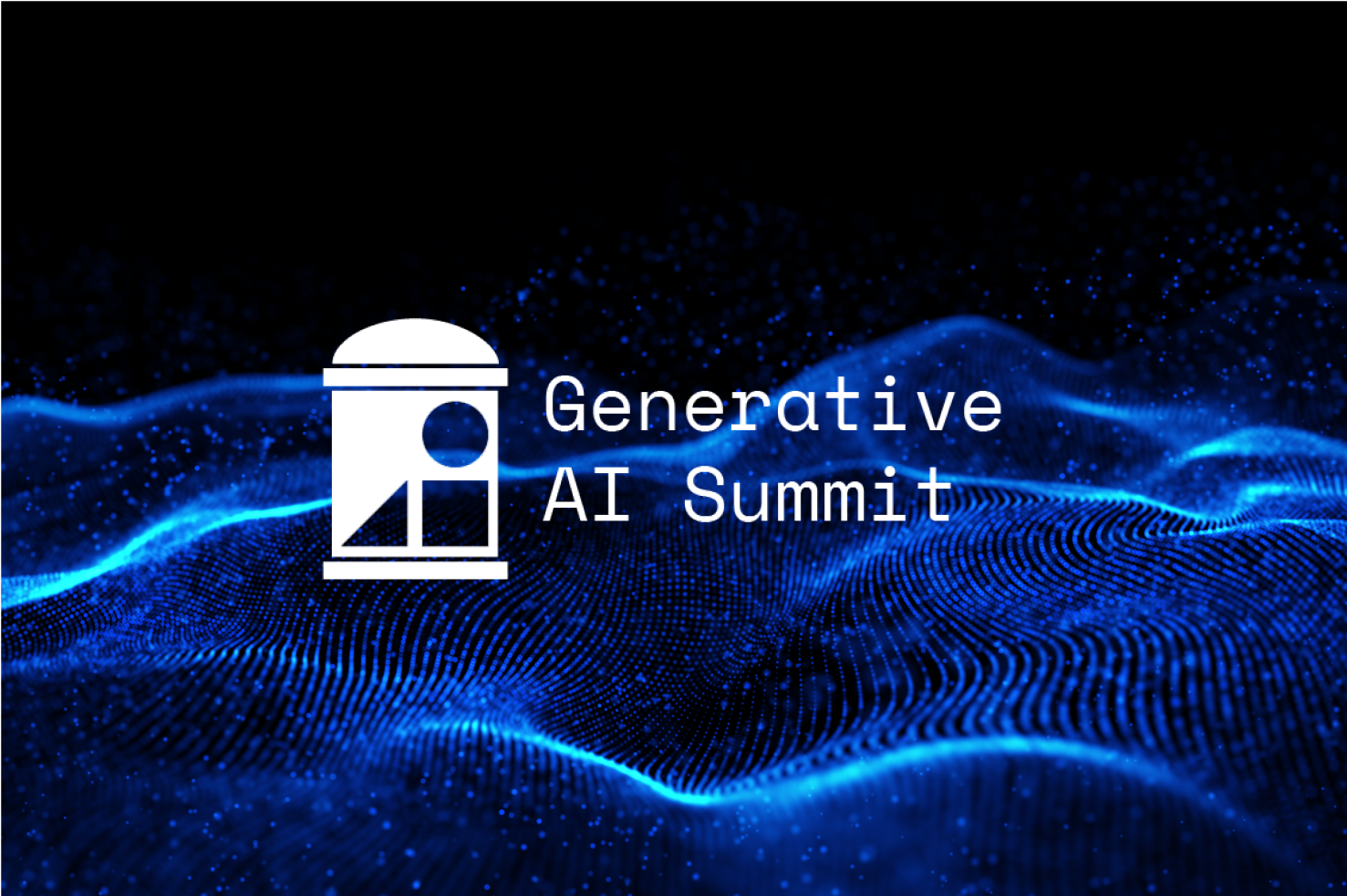
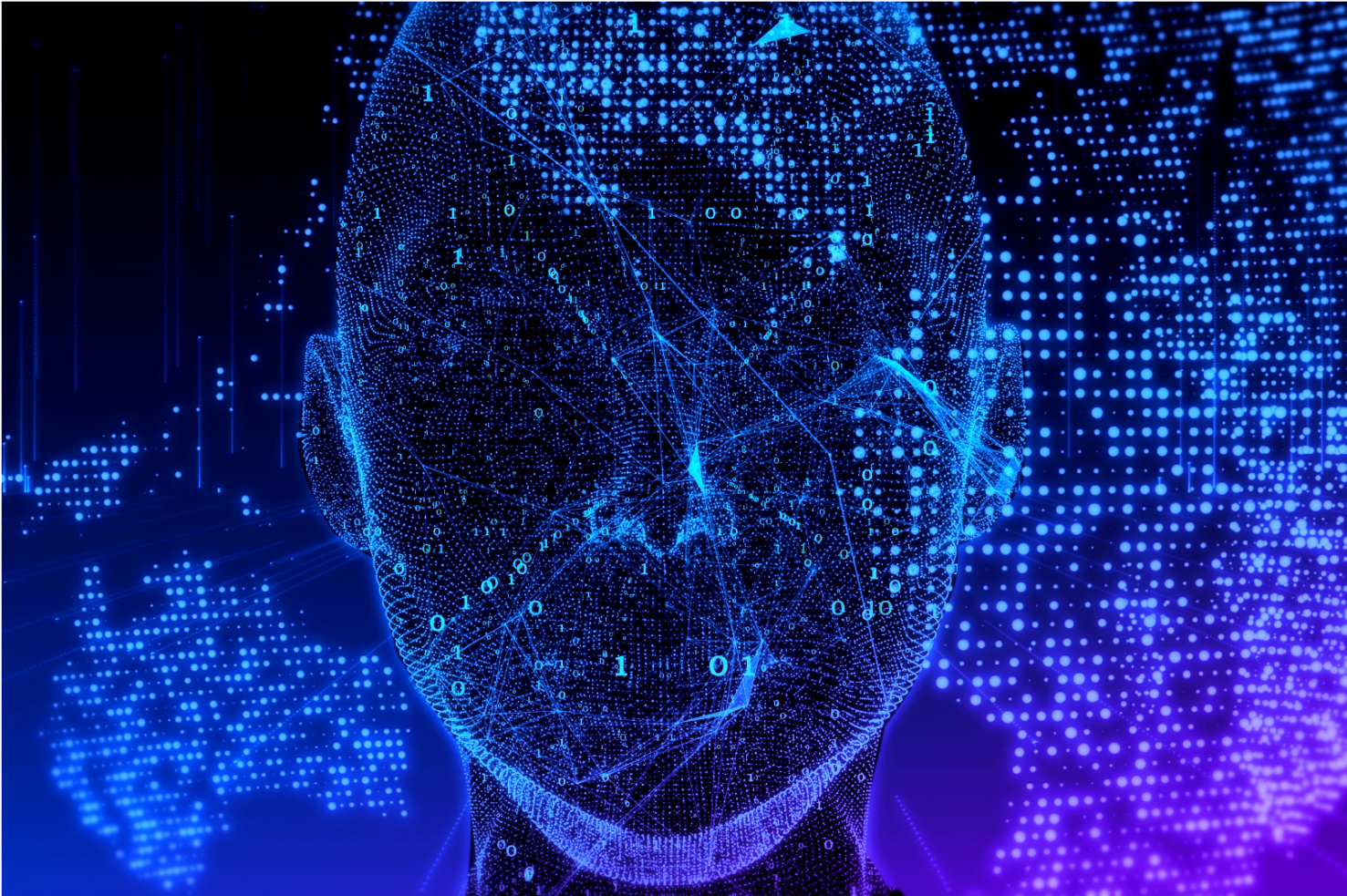
On March 3, 2023, MIT hosted the Inaugural v in Cambridge, MA, with Google’s former CEO Eric Schmidt delivering a keynote. In one of the panels, Catherine Havasi, Chief of Innovation and Technology Strategy at Babel Street, made another insightful analogy. She states that AI is also similar to the internet, explaining that in the 2000s it was identified as a standalone industry, separate from the others. Nowadays, every company, indistinct of industry, has incorporated the application of the internet (company website, e-commerce sales channel, customer acquisition tool, virtual meetings, et cetera) as part of their core strategy.
Similar to electricity and the internet, AI is a powerful tool to be embedded within a business model. AI is the key differentiator, the insurmountable moat to be constructed by board members, CEOs, and strategists. AI is best understood as a tool that is growing more and more essential for every business to possess. Artificial Intelligence is a once-in-a-century revolution that no one can afford to miss.

Sebastian Barriga
Investor, MIT Sloan & Visiting Fellow, DeltaV, YPO Member.
www.ypo.org